引用本文: |
-
陈观林,程钊,邹凌,杨武剑,李甜.基于BERT的危险化学品命名实体识别模型[J].广西科学,2023,30(1):43-51. [点击复制]
- CHEN Guanlin,CHENG Zhao,ZOU ling,YANG Wujian,LI Tian.Named Entity Recognition Model of Hazardous Chemicals Based on BERT[J].Guangxi Sciences,2023,30(1):43-51. [点击复制]
|
|
本文已被:浏览 283次 下载 559次 |
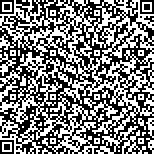 码上扫一扫! |
基于BERT的危险化学品命名实体识别模型 |
陈观林1,2, 程钊1,2, 邹凌2, 杨武剑1, 李甜1
|
|
(1.浙大城市学院, 计算机与计算科学学院, 浙江杭州 310015;2.常州大学计算机与人工智能学院, 江苏常州 213164) |
|
摘要: |
针对危险化学品实体识别及关系识别的问题,本文基于双向长短期记忆网络连接条件随机场(Bidirectional Long Short-Term Memory with Conditional Random Field,BiLSTM-CRF)模型,通过引入双向编码器表示(Bidirectional Encoder Representation from Transformers,BERT)模型结合多头自注意力机制,提出了一种预训练命名实体模型BERT-BiLSTM-self-Attention-CRF,通过对危险化学品的文本进行字符级别编码,得到基于上下文信息的字向量,增强了模型挖掘文本全局和局部特征的能力。实验结果表明,在自行构建的数据集上,本文模型优于其他传统模型,其F1值为94.57%。 |
关键词: 命名实体识别|深度学习|危险化学品|预训练模型|自注意力机制 |
DOI:10.13656/j.cnki.gxkx.20230308.005 |
|
基金项目:浙江省重点研发计划项目“危险化学品智慧监控及事故预防平台”(2020C03091)资助。 |
|
Named Entity Recognition Model of Hazardous Chemicals Based on BERT |
CHEN Guanlin1,2, CHENG Zhao1,2, ZOU ling2, YANG Wujian1, LI Tian1
|
(1.School of Computer and Computing Science, Hangzhou City University, Hangzhou, Zhejiang, 310015, China;2.School of Computer Science and Artificial Intelligence, Changzhou University, Changzhou, Jiangsu, 213164, China) |
Abstract: |
Aiming at the problems of hazardous chemicals entity recognition and relationship recognition,based on the Bidirectional Long Short-Term Memory with Conditional Random Field (BiLSTM-CRF) model,the pre-training named entity model BERT-BiLSTM-self-Attention-CRF is proposed by introducing the Bidirectional Encoder Representation from Transformers (BERT) model in combination with the multi-head self-attention mechanism.By encoding the text of hazardous chemicals at the character level,the character vector based on context information is obtained,which enhances the ability of the model to mine the global and local features of the text.The experimental results show that the proposed model is superior to other traditional models on the self-built data set,and its F1 value is 94.57%. |
Key words: named entity recognition|deep learning|hazardous chemicals|pre-training model|self-attention mechanism |