引用本文: |
-
葛丽娜,陈园园,周永权.密度峰值聚类算法研究现状与分析[J].广西科学,2022,29(2):277-286. [点击复制]
- GE Lina,CHEN Yuanyuan,ZHOU Yongquan.Research and Analysis of Adaptive Density Peak Clustering Algorithm[J].Guangxi Sciences,2022,29(2):277-286. [点击复制]
|
|
本文已被:浏览 497次 下载 638次 |
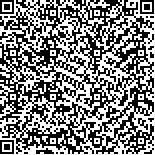 码上扫一扫! |
密度峰值聚类算法研究现状与分析 |
葛丽娜1,2,3, 陈园园1, 周永权1,3
|
|
(1.广西民族大学人工智能学院, 广西南宁 530006;2.广西民族大学, 网络通信工程重点实验室, 广西南宁 530006;3.广西混杂计算与集成电路设计分析重点实验室, 广西南宁 530006) |
|
摘要: |
密度峰值聚类(Clustering by Fast Search and Find of Density Peaks,DPC)算法是一种新型的基于密度的聚类算法,通过选取自身密度高且距离其他更高密度点较远的样本点作为聚类中心,再根据样本间的局部密度和距离进行聚类。一方面,虽然DPC算法参数唯一、简单、高效,但是其截断距离的取值是按经验策略设定,而截断距离值选取不当会导致局部密度和距离计算错误;另一方面,聚类中心的选取采用人机交互模式,对聚类结果的主观影响较大。针对DPC算法的这些缺陷,目前的改进方向主要有3个:改进截断距离的取值方式、改进局部密度和距离的计算方式以及改进聚类中心的选取方式。通过这3个方向的改进,使得DPC过程自适应。本文对DPC算法的自适应密度峰值聚类算法的研究现状进行比较分析,对进一步的工作进行展望并给出今后的研究方向:将DPC算法与智能算法有机结合实现算法自适应,对于算法处理高维数据集的性能也需要进一步探索。 |
关键词: 密度峰 聚类算法 自适应 截断距离 聚类中心 |
DOI:10.13656/j.cnki.gxkx.20220526.007 |
投稿时间:2021-06-11 |
基金项目:国家自然科学基金项目(61862007)和广西自然科学基金项目(2018GXNSFAA281269)资助。 |
|
Research and Analysis of Adaptive Density Peak Clustering Algorithm |
GE Lina1,2,3, CHEN Yuanyuan1, ZHOU Yongquan1,3
|
(1.School of Artificial Intelligence, Guangxi University for Nationalities, Nanning, Guangxi, 530006, China;2.Key Laboratory of Network Communication Engineering, Guangxi University for Nationalities, Nanning, Guangxi, 530006, China;3.Guangxi Key Laboratory of Hybrid Computation and IC Design Analysis, Nanning, Guangxi, 530006, China) |
Abstract: |
Clustering by fast search and find of density peak (DPC) is a new type of density-based clustering algorithm.It selects the sample points with high density and far from other higher density points as the clustering center,and then clustering is carried out according to the local density and distance between samples.Although the parameters of the DPC algorithm are unique,simple and efficient,the value of the cutoff distance is set according to the empirical strategy,and the improper selection of cutoff distance will lead to errors in the calculation of local density ρ and distance δ. On the other hand,the selection of clustering center adopts human-computer interaction mode,which has a great subjective influence on the clustering results.Aiming at these defects of DPC algorithm,there are three main improvement directions at present:improving the selection of cutoff distance,improving the calculation method of local density and distance,and improving the method of selecting cluster centers.Through the improvement of these three directions,the process of DPC is adaptive.Finally,the future work is prospected and the future research direction is given:DPC algorithm and intelligent algorithm are organically combined to realize algorithm adaptation,and the performance of the algorithm to deal with high-dimensional data sets needs to be further explored. |
Key words: density peak clustering algorithm adaptive cutoff distance clustering center |