引用本文: |
-
程小辉,李贺军,邓昀,陶小梅,黎辛晓.基于ME-ANet模型的糖尿病视网膜病变分级[J].广西科学,2022,29(2):249-259. [点击复制]
- CHENG Xiaohui,LI Hejun,DENG Yun,TAO Xiaomei,LI Xinxiao.Study on Grading of Diabetic Retinopathy Based on Me-ANet Model[J].Guangxi Sciences,2022,29(2):249-259. [点击复制]
|
|
本文已被:浏览 589次 下载 603次 |
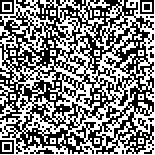 码上扫一扫! |
基于ME-ANet模型的糖尿病视网膜病变分级 |
程小辉1,2, 李贺军1,2, 邓昀1,2, 陶小梅1, 黎辛晓1,2
|
|
(1.桂林理工大学信息科学与工程学院, 广西桂林 541006;2.广西嵌入式技术与智能系统重点实验室, 广西桂林 541006) |
|
摘要: |
糖尿病视网膜病变(Diabetic Retinopathy,DR)是一种致盲率很高的眼科疾病。不同病变等级的视网膜图像之间差异小且病灶点分布无规律。针对现有深度模型对DR中的相似病灶点识别率低,严重影响模型分类精度的问题,本研究以深度学习为基础,构建新的模型架构进行训练,提出一种集成MobileNetV2和EfficientNetB0深度模型的注意力网络:ME-ANet。模型集成分为头部和主干两部分,将深度模型的浅层部分融合构成网络的头部,训练时采用迁移学习的策略对网络模型参数进行初始化,减少训练中的过拟合问题。主干部分利用上述两种模型的核心结构,设计3个阶段集成模块进行特征提取。同时设计全局注意力机制(Global Attention Mechanism,GAM)并分别嵌入到3个阶段的集成模块中。模型的改进加速了网络的收敛速度,该网络模型实现了对图像浅层信息的特征融合提取,减少了微病灶特征信息在训练过程中的卷积丢失问题,模型的分类精度进一步得到改善。通过模型集成构建特征提取主干网络,提高了模型对低级特征信息的学习,注意力机制抑制非病变特征信息,强化典型病灶特征学习,从而实现细粒度分类,进一步提升了模型的分类性能。 |
关键词: 糖尿病视网膜病变 迁移学习 集成模块 注意力机制 特征融合 |
DOI:10.13656/j.cnki.gxkx.20220526.004 |
投稿时间:2022-02-13 |
基金项目:国家自然科学基金项目(61906051),广西自然科学基金项目(2018GXNSFAA281235)和广西中青年教师基础能力提升项目(2018KY0248,2020KY06026)资助。 |
|
Study on Grading of Diabetic Retinopathy Based on Me-ANet Model |
CHENG Xiaohui1,2, LI Hejun1,2, DENG Yun1,2, TAO Xiaomei1, LI Xinxiao1,2
|
(1.College of Information Science and Engineering, Guilin University of Technology, Guilin, Guangxi, 541006, China;2.Guangxi Key Laboratory of Embedded Technology and Intelligent System, Guilin, Guangxi, 541006, China) |
Abstract: |
Diabetic retinopathy (DR) is an ophthalmic disease with a high blindness rate.The difference between retinal images of different pathological grades was small and the distribution of lesion points was irregular.Aiming at the problem that the existing depth model has low recognition rate for similar lesions in DR,which seriously affects the classification accuracy of the model.This study builds a new model architecture for training based on deep learning,and proposes an attention network that integrates MobileNetV2 and EfficientNetB0 depth models: ME-ANet.The model integration is divided into two parts:head and backbone,and the shallow part of the depth model is fused to form the head of the network.During the training,the transfer learning strategy is used to initialize the network model parameters to reduce the over-fitting problem in the training.The backbone part uses the core structure of the above two models to design a 3-stage integrated module for feature extraction.At the same time,the Global Attention Mechanism (GAM) is designed and embedded into the 3-stage integrated module respectively.The improvement of the model accelerates the convergence speed of the network.The network model achieves feature fusion extraction of shallow image information,reduces the problem of convolution loss of micro lesion feature information during the training,and further improves the classification accuracy of the model.The feature extraction backbone network is constructed through model integration,which improves the model's learning of low-level feature information,the attention mechanism suppresses non-lesion feature information,and strengthens the learning of typical lesion features,thereby realizing fine-grained classification and further improving the classification performance of the model. |
Key words: diabetic retinopathy transfer learning integrated module attention mechanism feature fusion |